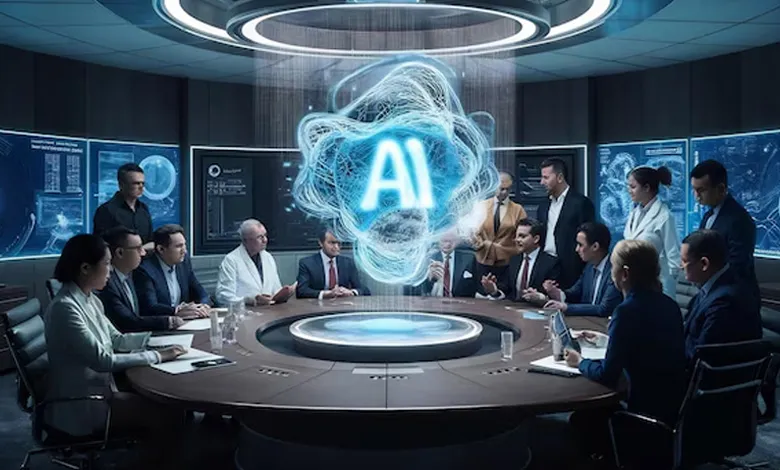
Researchers have identified 32 best practices to address a significant crisis in the scientific community. There is a great difficulty in the machine learning of the AI system, which is by no means limited to the production of multiple claims across various fields. Such a matter is undermining the authority of scientific research and tampering with this field as a whole.
AI can help doctors spot early indications and policymakers achieve peace. The scientific community is questioning the accuracy and effectiveness of the machine learning application as more cases show severe flaws. This issue has alarmed the scientific community, and perhaps 10,000 manuscripts are under investigation.
A team of 19 interdisciplinary researchers, led by Princeton University computer scientists Arvind Narayanan and Sayash Kapoor, has released recommendations for machine learning in scientific research design.
Arvind Narayanan, a computer science professor and head of Princeton’s Center for Information Technology Policy, discussed the challenges of transitioning from traditional statistical methods to machine learning systems. He stated that, while machine learning systems demand significantly more labor to eliminate errors, some of these errors may occur without an intervention to enhance the standards of scientific and reporting norms. As a result, the scientific community risks having different fields experience identical crises over and over.
In his presentation, Arvind Narayanan explained how machine learning is already widely used in all sciences with no standards in place to ensure that the methods and algorithms are error-free. He warned that this predicament, which he dubbed a reproduction crisis, could have far-reaching consequences beyond the replication problem that Social Psychology is currently experiencing. This is because, unlike the replication crisis, the reproduction issue lacks safeguards across scientific areas.
According to Kapoor, a graduate student who collaborates with Narayanan, a systemic solution is necessary to address the systemic problem. He oversaw the collaborative effort that resulted in developing the new consensus criteria.
The checklist is dedicated to ensuring the accuracy of the research using machine learning. Science recognizes the possibility of independently repeating an experiment and validating claims. This capability is rather the foundation for all future developments. Unlike earlier checklists, which largely address discipline-specific difficulties, particularly in medicine, the new set of guidelines begins with the core procedures and extends their applicability to all quantitative disciplines.
Transparency may be identified as a significant consequence. Researchers must explain each machine learning model’s code, training and testing data, hardware specs, experimental design, project objectives, and study restrictions. The system can be applied to private datasets and sophisticated hardware settings, as the authors noted.
The recommendations were to be basic enough for widespread use but specific enough to catch and consider major faults.
The guidelines will help researchers improve their work, and peer reviewers can use the checklist to evaluate scholarly publications. Journals could need the standard to publish articles.
According to Arvind Narayanan, scientific literature frequently contains avoidable errors, especially in applied machine learning research. He further stated that our endeavors aim to assist researchers in substantiating the validity of their findings and furnish them with the requisite regulatory frameworks.