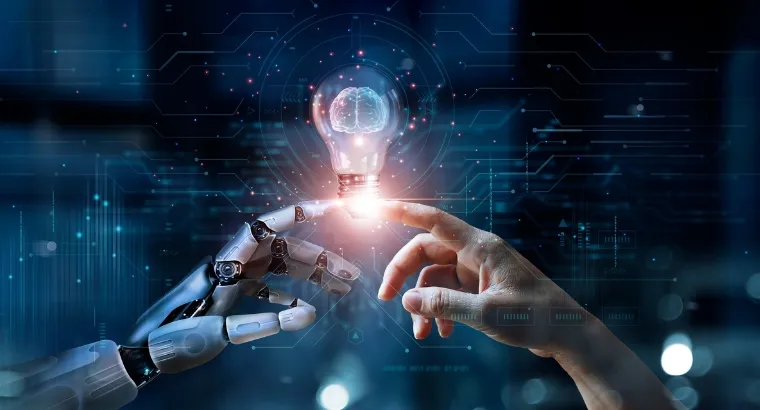
Utilizing AI, scientists at the MIT Lincoln Laboratory are obtaining a clearer image of the atmospheric layer closest to the Earth’s surface. Their methods may enhance the ability to forecast weather and drought.
The planetary boundary layer (PBL), being a core component of the troposphere, notably just above the Earth’s surface, holds a key position in regulating our temperature and weather patterns. According to the 2018 Planetary Science Decadal Survey, this level, which is the area where the earth’s surface either absorbs, releases, or exchanges with moisture and atmospheric temperature, is an essential element in understanding extreme weather and climate change.
Adam Milstein, a member of Lincoln Laboratory’s Applied Space Systems Group, emphasizes how PBL directly impacts aerosol dispersion and human health due to the easy mixing of these aerosols into the atmosphere.
Understanding PBL is a critical requirement for improving storm forecasting and climate projections. Nonetheless, precisely distinguishing its height may be a problem due to the existing level of technology. For four years, the scientists at the Lincoln Laboratory have been working tirelessly to investigate the PBL using cutting-edge technologies, such as machine learning, to create three-dimensional atmospheric profiles and help in drought prediction.
The laboratory has developed rapid and effective neural network algorithms for NASA missions, such as Time-Resolved Observations of Precipitation Structure and Storm Intensity with a Constellation of Smallsats (TROPICS) and Aqua, with over ten years of experience.
The algorithms that process temperature and humidity data from satellite instruments have greatly improved the observed data accuracy and global coverage. The data is utilized by TROPICS for storm analysis that is conducted in near-real-time. On the other hand, Aqua has expanded its functionalities to encompass drought monitoring, fire prediction, and forecasting models.
In the beginning, relying on faster and simpler “shallow” neural network models, companies have found that more sophisticated “deep” learning techniques are necessary for a more complete exploration of the PBL. These complicated models treat the atmospheric data as a three-dimensional image, thereby providing a refined statistical representation and enhancing the accuracy of data interpretation.
Recent developments in conjunction with NASA’s Joseph Santanello and William Blackwell from Lincoln Laboratory have unveiled remarkable progress in the precise characterization of the planetary boundary layer (PBL). This holds significant relevance in the comprehension and anticipation of climate phenomena, specifically droughts.
As a result, a recently published Global Drought Snapshot report emphasized the need to deal with droughts all over the world. It explained that surface-level humidity, especially within the PBL, is a primary indicator of drought conditions. Previously, monitoring soil humidity was the primary purpose of remote sensing technologies. However, recent research has focused on the atmosphere to generate more precise drought forecasts.
Milstein, in conjunction with Michael Pieper of the Lincoln Lab and NASA Jet Propulsion Lab, is using these neural network models to boost the drought forecast in the continental USA. By combining the application of the new deep learning techniques from the Aqua mission with the established workflow, they aim to achieve the greatest progress towards working on drought forecasts.
With the decadal experience of partnering with NASA on Earth observation research, Milstein expresses the incredible amount of knowledge and the scientific hurdles along the way. Another step in their investigation is to verify their deep learning models against data from radiosondes, i.e., weather balloons with measuring devices, to make sure the accuracy of their predictions is good.
Milstein emphasized the possibility of the neural network method outperforming the existing features in the overall approach to drought prediction and being a sustainable technique that scientists will use in the future.